The popularity of commercial Generative AI models, like ChatGPT, Claude, and Gemini, has caused businesses to look for ways to implement AI into their operations.
They recognize that artificial intelligence (AI) can help streamline operations, make faster business decisions, and sift through data in a fraction of the time it normally takes.
More than 80% of companies plan to embrace AI automation by 2025, while 33% of businesses already use it.
As businesses embrace predictive models and machine learning algorithms, serious questions begin to arise:
- Can generative AI models be trusted?
- Can they produce biased results?
- How accurate is the data they output?
- What other alternatives could have been chosen?
- Is our data and the data of our customers safe?
- How do we know if the output is the best option for us?
Making mission-critical decisions for your business using the output from an AI model requires one thing:
Trust.
If you’re going to change how you make decisions, interact with customers, and run your business based on what AI tells you, you need to trust the model.
That’s where Explainable AI comes in.
What Is Explainable AI?
Generative AI models are often considered black boxes that are impossible to interpret.
A user inputs a prompt, the AI model reaches some valuable conclusion — finding qualified leads, writing compelling content, creating sales predictions based on previous trends — and the user is left with the option to take it or leave it.
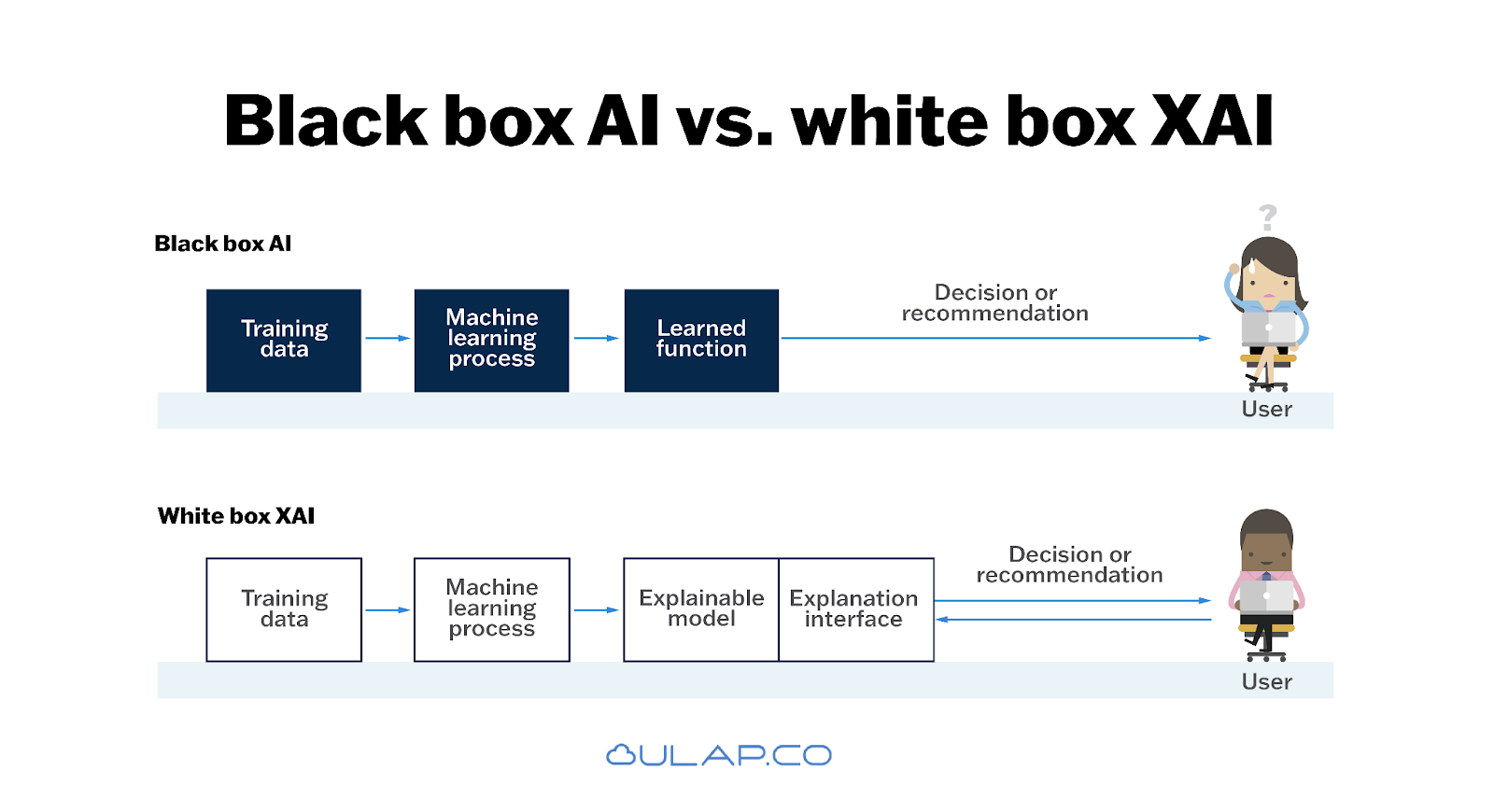
No insight was provided into how the AI model generated the output it did, what data the model pulled from, nor how confident it was in that decision.
Explainable AI combats that lack of transparency by giving users visibility into all aspects of the AI model.
It opens the black box of Generative AI by helping users understand the machine learning (ML), deep learning, and neural network algorithms that drive the AI model.
The folks over at Perplexity have introduced several XAI concepts into their publicly available application Here’s what it looks like in action:
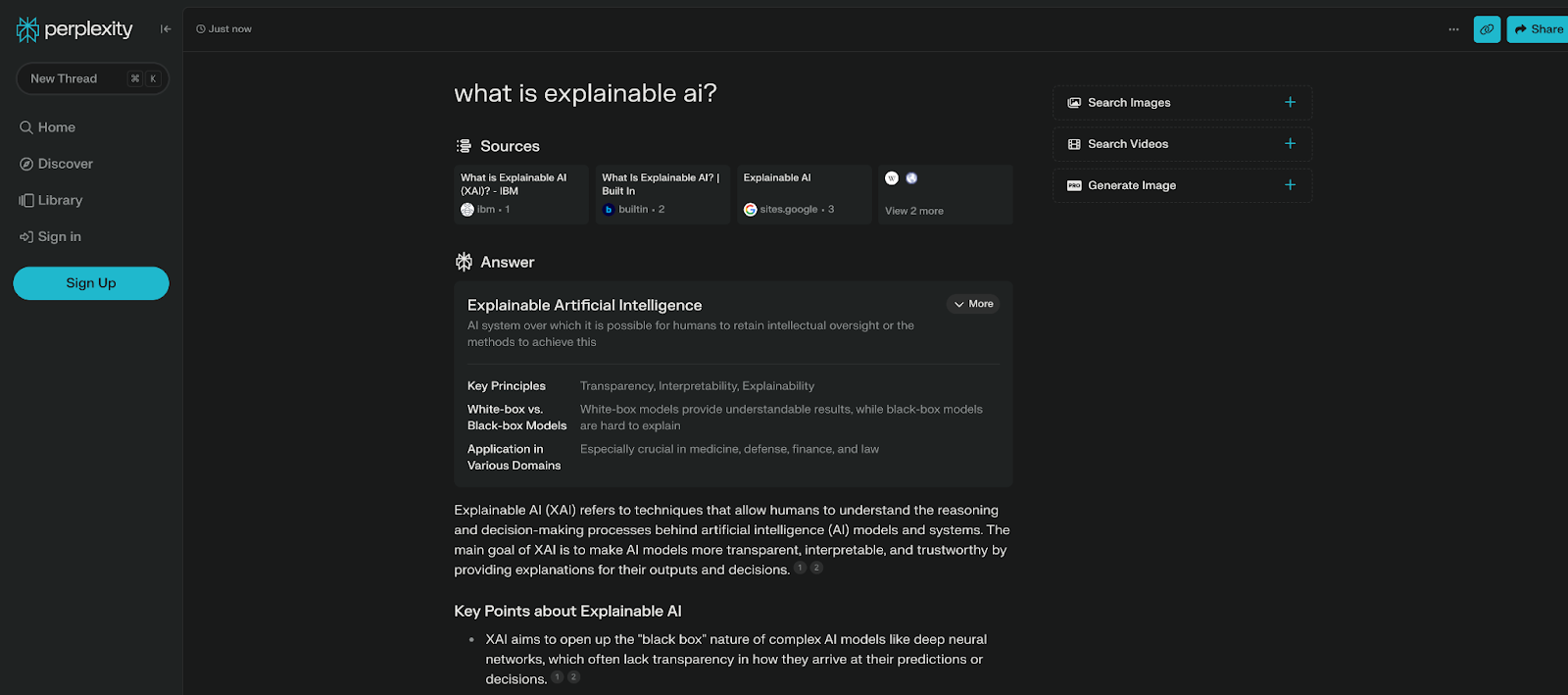
Perplexity provides users with the sources that are used to provide the answer. Additionally, footnotes are included within the answer that directly link users to the source. The explanation provides context to the output, which makes it easier for the user to understand and trust the model’s decision.
Why Explainable AI Matters
Generative AI models have their limitations:
- Bias based on race, gender, age, or location
- Model performance degradation due to production data and training data differences
- Inaccurate, copyrighted, or morally different data in the training set
- Hallucinations when the model doesn’t know the answer
These limitations and more can make it hard to trust AI—and dangerous to trust it blindly with mission-critical business decisions.
Explainable AI provides users with seven key areas for understanding AI, including:
- Transparency: do stakeholders understand the model’s decisions in terms of formats and language
- Causality: do the predicted changes in the output due to input perturbations also occur in the actual system?
- Privacy: can the protection of sensitive user information be guaranteed?
- Fairness: can it be verified that model decisions are fair over protected groups?
- Trust: how confident are the human users in working with the system?
- Usability: How capable is the system of providing a safe and effective environment for users to perform their tasks?
- Reliability: how robust is the system performance against changes in parameters and inputs?

When it comes to the model output, the user does not only have the option to take it or leave it. Instead, the user can dissect the context behind the output to make a more profound and better decision for their business.
Four Ways Explainable AI Can Benefit Your Business
This leads to a burning question: how can explainable AI benefit my business?
Businesses that implement Explainable AI into their operations, as opposed to Generative AI, see four main benefits.
Increasing Productivity
Monitoring and maintaining AI systems can be complicated, especially when models are not built on an extensive MLOps system.
The techniques behind Explainable AI can speed up the process of revealing errors and areas for improvement, allowing your MLOps team to monitor and maintain your systems more efficiently.
Building User Trust and Adoption
Explainability enables users to trust AI models.
As discussed throughout this article, when users know why a model makes its prediction, recommendation, or output, they are more likely to trust that output.
Think of it this way.
When working with an expert, it’s easier to trust the recommendations they give when they explain WHY that recommendation is right for you.
It’s no different with AI. When the model tells you WHY it gave you an output, it’s easier to follow its logic and trust its recommendation.
And the more people trust AI, the more likely they are to use it, which should be the goal.
Surfacing New Problem Interventions
In many cases, a deeper understanding of the why of an AI prediction can be even more valuable than the prediction or recommendation itself.
Let’s say you’re using AI to determine which of your clients is likely to churn in Q4.
Generative AI will give you a list of clients, which is helpful, but explainable AI will give you that same list, plus the traits and indicators that helped the model reach that conclusion.
This means you’ll have the information needed to help prevent client churn from occurring, which is far more valuable to a business leader than a prediction of churn alone.
Mitigating Regulatory and Other Risks
Explainability helps businesses mitigate risks.
Legal and risk teams can use the AI model's explanation and the intended business use case to confirm the system complies with applicable laws and regulations.
In some cases, explainability is a requirement — or will become one soon.
The California Department of Insurance requires insurers to explain adverse actions taken based on complex algorithms, while a new draft of the EU AI regulation contains specific explainability compliance steps.
Adopting Explainable AI helps mitigate those risks and ensures your business complies with upcoming regulations.
Use Cases for Explainable AI
So, what does Explainable AI look like in the field?
Here are a few examples of how businesses use Explainable AI to improve their operations, safety, and decision-making.
Financial Services:
Financial services companies (think banks, financial planners, investors, and the like) are using Explainable AI to:
- Improve customer experiences with a transparent loan and credit approval process
- Provide explanations for detecting fraudulent activities
- Educating their customers on how to make financial decisions that align with their goals
- Preventing bias, especially based on race, gender, age, and ethnicity, in financial decisions
- Explain the reasoning behind an investment recommendation
The explanations of why the model generated an output also help them comply with local and federal laws.
Smart money is starting to move with Explainable AI.
Cyber Security :
Likewise, cyber security businesses and teams are using Explainable AI to improve their operations.
Cyber teams are often faced with alert fatigue.
Cyber operations centers heavily depend on technologies that produce many reports, logs, and alerts—all of which the team needs to review.
As a result, many alerts are muted and never reviewed by cyber analysts, or the threat isn’t correctly labeled by network monitoring technologies.
That’s where teams are turning to Explainable AI.
The AI model can quickly explain why an alert surfaced and why it was important and give a rating of its confidence in the alert.
These explanations make it easier for a typically understaffed cyber team to quickly analyze and respond to threats.
Secure, Transparent Communications:
The rise of Generative AI has produced an unforeseen consequence:
Your data and conversations are not always secure.
Take Slack, for instance.
The communications company has come under scrutiny for its sneaky AI training methods.
According to TechCrunch, the company is “using customer data specifically to train ‘global models,’ which Slack uses to power channel and emoji recommendations and search results.”
Or Microsoft’s Copilot.
According to Simon Pardo, director of technology specialists Computer Care, “Copilot+ Recall takes screenshots of a user’s computer activity and analyses them with AI to let users search through their past activities, including files, photos, emails, and browsing history.”
Imagine the security risks that businesses face here.
Sensitive and private information is being collected and consolidated into one location, exposing a vast amount of information if the system is hacked.
That doesn’t even take into account how companies like Slack and Microsoft are profiting from user conversations.
That’s why Ulap is working with a partner to develop a communication platform that enables secure and trustworthy conversations that are not recorded and used for product development.
Privacy is protected and user conversations are not being exploited for profit.
All of that is being built on the back of Explainable AI, which spins up ephemeral algorithms and manages how resources are used — with one core purpose:
Enable secure, transparent communications where your conversations are safe.
Commercial Real Estate:
Even commercial real estate investors and developers are jumping on the Explainable AI bandwagon.
Companies are using Explainable AI to:
- Understand why a certain site will be best for their development project
- Get a score for each property based on the project criteria
- Research the impact on traffic patterns, utilities, and property value and what can be done to avoid a negative impact
- Prepare presentations and proposals that give a clear explanation as to the pros and cons of each property
What Makes Explainability Challenging
Developing the capacity to make AI explainable is challenging.
Doing so requires a deep understanding of how the AI model works and the data types used to train it. This may seem simple, but the more sophisticated an AI model becomes, the harder it is to pinpoint how it came to a particular output.
AI models get ‘smarter’ over time — they ingest data, gauge the power of different algorithms, and update the existing model all within minutes or even fractions of a second. The more the AI model learns, the harder it becomes to follow the insight audit trail.
Think of it this way.
Why did you choose to wear the shirt you wore today? Was it the weather, your mood, what was clean and available, what you would be doing that day…or a mix of all of them?
It’s not so easy to follow that insight trail.
Now imagine trying to follow the insight trail of a more complicated task — like reviewing a thousand rows on an excel spreadsheet to find the pattern of your clients and predict which are likely to churn.
That is why Explainability is challenging to implement.
How Businesses Can Make AI Explainable
Building a framework for explainability allows businesses to capture AI's full value and stay ahead of its advancements.
This framework should govern the development and usage of AI within your company to ensure accuracy, security, and compliance with industry and government regulations.
AI Governance Committee:
Establishing an AI governance committee is the first step in making AI explainable.
The role of this committee should be to establish:
- Responsible AI guidelines
- A risk taxonomy to classify different AI use cases
- A process for model development teams to assess each use case
- Tracking of assessments within a central inventory
- Monitor AI systems for compliance with legal requirements and adherence to responsible AI principles
Your AI governance committee will need to consider the level of explainability to adhere to — are basic explainability requirements enough, or do you need to go beyond them to achieve greater trust, adoption, and productivity?
Think of it this way.
Simplifying an AI model’s mechanics might improve user trust but could make the model less accurate.
Which is more important to your company and to your specific use case?
Your AI governance committee should determine those priorities to guide your team in developing AI models.
Responsible AI Guidelines:
One of the first tasks of your AI governance team should be to make explainability part of your responsible AI guidelines.
Your company values are not enough. As your business embraces and uses AI in your regular operations, a set of guidelines can prevent unintentional but significant damage to your brand reputation, workers, individuals, and society.
Responsible AI guidelines should include:
- Appropriate data acquisition
- Data-set suitability
- Fairness of AI outputs
- Regulatory compliance and engagement
- Explainability
Invest in the right tech, people, and tools:
Lastly, you’ll need to make the right investments to stay ahead of technological and legal changes in AI explainability.
The Right People:
Develop a talent strategy to hire and train the right people for your explainable AI needs. Consider:
- Legal and risk talent who can engage with both the business and technologists
- Technologists who are familiar with the legal issues or focused on technology ethics
- Talent with diverse points of view to test whether the explanations are intuitive and effective for different audiences
Hiring the right people makes all the difference in ensuring the development of the right explainability for your AI models.
The Right Tech & Tools:
Ensure you acquire the right tech and tools for meeting the needs identified by your development team.
A commercial LLM or an off-the-shelf solution may be cheaper upfront but not the right solution for your AI tool.
For example, some explainability tools rely on post-hoc explanations that deduce the relevant factors based only on a review of the system output — which can lead to a less-than-accurate explanation of the factors that drove the outcome.
Review the explainability requirements of your AI use cases to ensure you invest in the right tech.
Although the up-front cost of bespoke solutions may be higher, it sometimes pays off in the long run because they can take into account the context in which the model is being deployed, including the intended users and any legal or regulatory requirements.
Find the Right Explainable AI Partner
Implementing Explainable AI into your business requires the right partner — someone to guide you through decisions that will shape your AI model.
Your explainable AI partner should help you:
- Find a solution that avoids vendor lock-in, allowing you to move your model when necessary.
- Select the appropriate open-source technology to build on
- Select the right licensing to ensure your model operates correctly
- Select the appropriate LLM for your needs
- Develops your model or modifies your model to introduce explainable AI
Ulap has guided commercial organizations and government agencies to selecting the right people, tools, and tech to implement Explainable AI into their models.
Schedule a time to see how we can help you do the same.